Do you remember the last time the power went off? At your home – you may have thought about the food that may go bad because the fridge didn’t work anymore. You probably checked closely your battery percentage on your phone to estimate how much it will last. Of course there's no TV, no internet, your laptop is also on battery and probably no entertainment system either. Maybe it's time to read that book you bought?
That's how dependent we are. We need electricity. We need to know it's there for us, at every minute of our day to day life; it's part of our life and we don't realize it until it's turned off for a while. If electricity is so important for personal use like yours and mine, can we just agree upon the importance in business areas and society nowadays? |
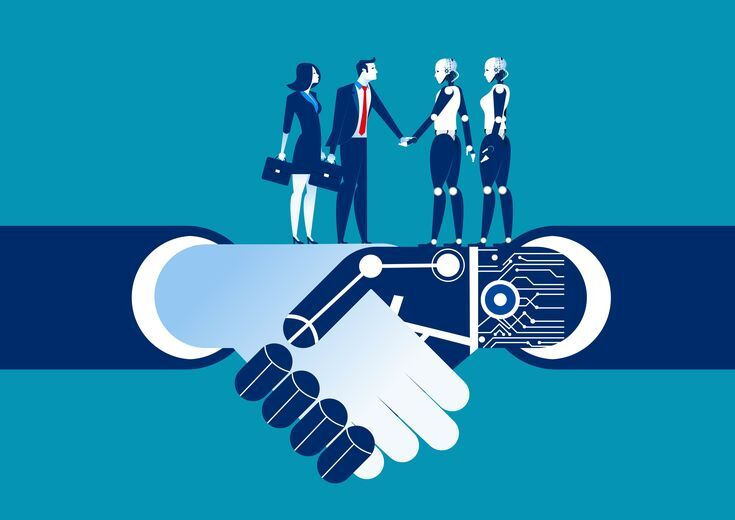
AI is the new electricity… in business operations
The first part of this quote is often attributed to Andrew Ng and possibly first published in 2017. The prominent figure in AI was surely considering all the implications back then, but the idea shared is mainly concentrating on how electricity revolutionized industries in the past and how AI has the potential to do the same.
Electricity is a foundational utility today and AI will become a fundamental infrastructure – that's the gist of it. This is a simple analogy that modern human beings can easily understand. Electricity has given us comfort in our lives and improved many aspects in the last 100 years. All the heavy lifting that AI is doing on the operational side of a business is also for our comfort, both employee and customer. This has seen improvements in the last seven years and as long as there's demand for it, it will keep on growing
Terminology can enrich your imagination but the term “AI” can be very vague. It's often used to refer to anything that mimics intelligence. The application of artificial intelligence can be present anywhere there's a decision making process. Dr. Hannah Fry has covered in her book Hello World: Being Human in the Age of Algorithms various use-cases of artificial intelligence in society and the impact on human life. Some scenarios are towards human assistance, such as flu outbreak prediction; other scenarios are heavily biased, such as recruitment based on historical data.
From another perspective, it’s worth pointing out that in late 1990, Whirlpool announced their “AI'' washing machines. These were implemented with fuzzy logic algorithms that adjusted the washing parameters such as the water level, the temperature and the RPM based on the load size and the type of fabric. This was also a step forward towards convenience.
An algorithm is a procedure to solve a problem. With AI, there are two key phrases widely used: training and inference (or prediction). The complexity of an AI system can grow, both in inference and training. There's always an algorithm at the core of these pipelines that processes the input and returns and output, but before it reaches that point (and very often, after it delivers the output), there are numerous steps to pre-process, to fine-tune and to fill in additional data.
In the AI landscape, a third crucial element emerges alongside the algorithm: the dataset. That's what we've been missing in the last decades. The idea of training a computer to make a decision about anything has been in our mind since 1950, but only in the last 10 years or less the missing puzzle was truly handled properly – companies have been gathering data and metadata so that eventually such a system can be built. Have they done it? Yes. But does it actually work?
As consumers, we sometimes know when we're dealing with AI in the sense of speaking or interacting with a robot; but we're not even close to actually knowing how much that company relies on AI.
From data analytics to understand the user's behaviour on a purchase flow; assess risks and detect anomalies to mitigate potential loss; and the well-known today customer service chatbot support. But that’s not all. It can gather data about tasks and the steps that are required to perform a task and then that data can be used to support the decision, be it to automate some parts of the routine or be it to train a system to be autonomous.
AI as a dependency in business operations
When a company decides to include AI in their business as usual, it's mostly a decision based on numbers, not on hype. While there are numerous services that sell the idea that AI can help, it's mainly a need to optimize costs somewhere in the operational side. From this perspective, AI is an investment, if done right.
The lack of electricity can slow down or even stall the activity in a company. It’s a dependency and in order to be fully operational, all dependencies must be available and work properly. With this idea in mind, what’s the cost of an AI power outage?
When Cloud companies arose, especially during the 2010s, they populated this idea of availability zones. It means having multiple data centers across the region – the country, the continent or the entire globe – so that it can mitigate and prevent a denial of service in case of a power outage and other types of disasters.
Cloud can and is currently being used as infrastructure for AI systems to ensure high availability and provide you with access to that environment, so your business operations can still perform. That’s just one point. The second point is to consider the complexity of an AI and its two major requirements: the algorithm and the dataset. Access to an electronic system means to be able to run that code, that algorithm. An AI system is highly sensible to what that it outputs based on its trained model. In other words, if your trained model is not available or not efficient, then you're in an AI blackout.
While there’s no solution for all cases, there are strategies for companies to recover fast and stay in business. Backups have been the number one strategy for decades. Backup your code, backup your database and if something bad happens then just deploy on a different machine and restore your data. Does it work with AI as well? Not in all cases.
If you're using AI in a critical decision making process, then your model must be up-to-date with the latest happenings in the world, such as politics, legislations, rules, but also company announcements, service offers – then a backup is only half the solution. What you also need is a way to quickly restore your prediction and training pipelines. For this to work you need qualified human personnel that can act fast and with precision. Today there’s a blurry line between the responsibilities of all engineers in this field, but this will change soon.
AI is here to stay. Be it the new electricity or the new internet or just the new system on the block that does the heavy lifting for you. The number of companies that use AI on the operational side will continue to grow and, with this adoption, a new need for human skills will develop. The job market will see new titles, new roles, new responsibilities, just as cloud has introduced DevOps. It will take a while to fully understand what's the description of that job, but it's getting there.
Let me close this article with some questions as food for thought – do you know how to transform your workforce today? Are you hiring the right personnel to face the AI landscape? How many humans do you have in the loop in this decision making process? Let’s talk about it.
// References
Fry, H. (2018). Hello World – How to be Human in the Age of the Machine.
The Wall Street Journal. (2017). Andrew Ng: AI Is the New Electricity.
// Alexandru Catrina // APRIL 12, 2024
Comments